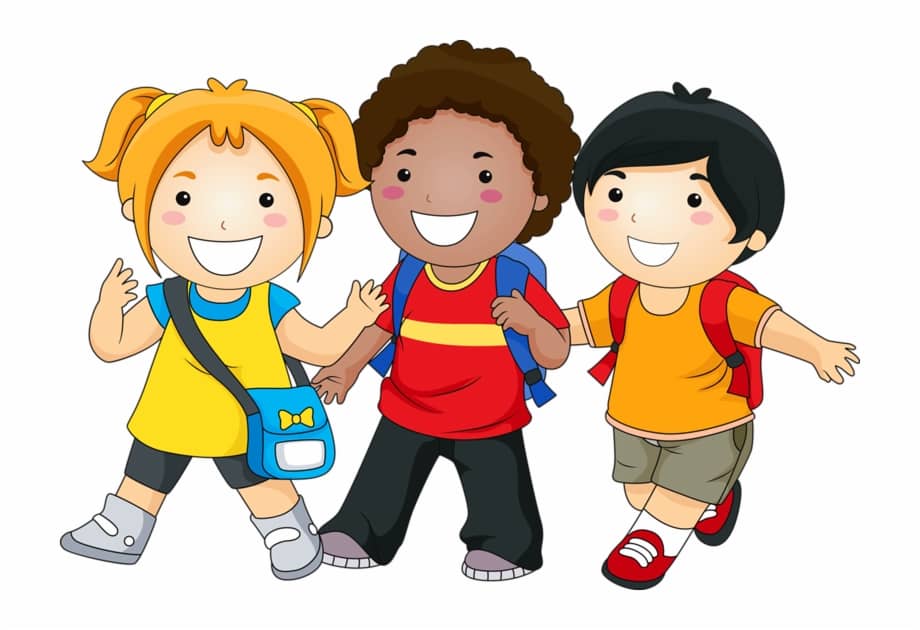
We are back with another installment of the Best Friend Series at the The P-Zero PM.
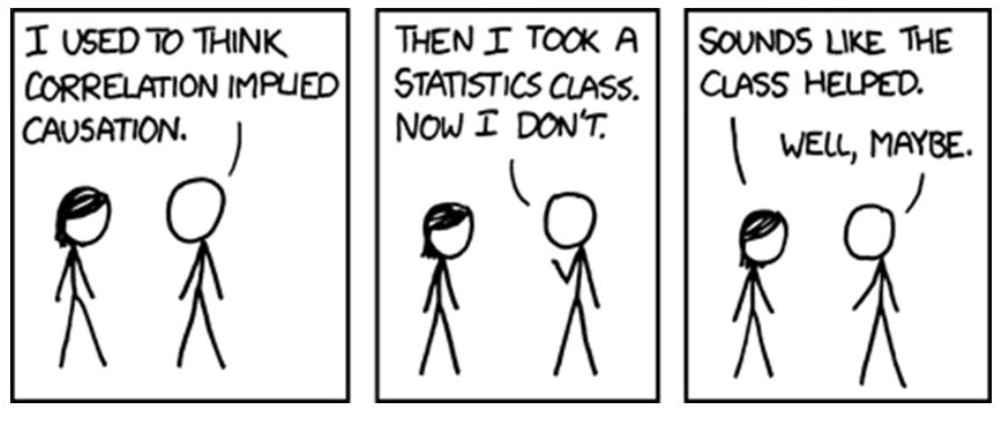
Correlation, causation, confounding variables, confidence interval…confused? These are terms that will become more familiar as you interact with your next best friend…The Data Scientist!
By the way, I love alliteration, in case you didn’t directly deduce that in the previous sentence.
Am I right?
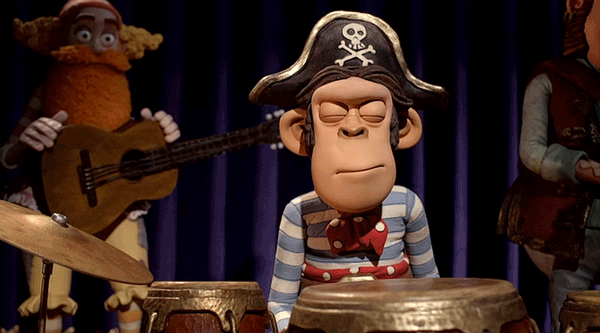
Ha! I’m here all night…leave the tips at the door!
Alright, back to the article…
Now, I know I have said that one of the most underrated best friends is the UX Researcher, but if there was a best friend that was a CLOSE second in terms of being underrated, it would be the Data Scientist. I say this because when people speak about the role of the data scientist, they usually relegate them to the stakeholders who simply take in data requests and send the requested data to the requester of said data. It’s as if data scientists simply service data requests like Lucille Ball on an assembly line trying to package chocolate coming down at an ever increasing speed.
I’m here to tell you that the data scientist is not ONLY here to service your data metric requests. Your data scientist is as much of a discoverer of information and problems as you are. They help turn a daunting amount of data into actionable and understandable insight. Additionally, your data scientist is the ruler of the infrastructure that supports the collection and monitoring of the data (i.e., the infrastructure that enables you to track your metrics). So, what are all the ways the data scientist (aka your new best friend) supports the entire product development process?
How does the Data Scientist support the product development process?
- Validating the extent to which a problem currently exists – In the Why Your UX Researcher is Your Best Friend article, I detailed how the UX researcher assists the product manager in validating or refuting a product hypothesis through research studies. Your data scientist can also provide data to assist you in the validation/refutation process by letting you know how prevalent a problem is on the platform for your users. For example, let’s say that you expect a specific subset of your user base (e.g., users in Australia) is not using a specific feature you created for the entire member-base. You have a potential solution that will encourage the use of this feature by your Australian demographic but want to be 100% sure this problem explicitly affects the Australian demographic. Your data scientist can uncover usage for the specific demographic you requested and, in their investigation, can perhaps uncover other demographics exhibiting the same behavior of your Australian userbase.
- Product Metric validation and consultation (Pre-Launch) – You’ve uncovered a problem and validated the need for a solution. You have mapped out a preliminary solution and its associated metrics. You have also worked with your engineers and designers to finalize what the actual solution will look like and how it will operate. At this point, you can (and should) consult your data scientist to answer the following questions:
- Are there any downstream metric effects that could occur after your feature is implemented? I.e., could your feature positively or negatively affect other core metrics or metrics of other teams’ products? As a new PM to the team, you won’t have a complete picture of the product ecosystem, so it’s best that you consult your data scientist to ensure you understand all of the potential downstream effects of your product.
- Are these the right metrics to even track? Just like the PM incorporates the expertise of the UX designer to finalize the product design and the expertise of the engineer to develop the technical infrastructure that will support the product, the PM should incorporate the expertise of the data scientist to ensure the metrics he/she will track are correct. It’s very possible there are other data contexts that may be missing in your analysis which would aid in determining the correct metrics to track. Additionally, the PM should confirm north star metrics and sign-post metrics (metrics that track your progress to potentially reaching your north star metric) with the data scientist. For more information on north star vs sign-post metrics, check out this article by Carl Anderson.
- Are these metrics actually measurable by the current infrastructure? If they’re not measurable directly, perhaps there’s a proxy metric to select that will tell the same story. If time permits, then perhaps your data scientist can set up the infrastructure to directly track the metric you desire.
- What is the pre-launch metric snapshot? Once you have finalized the metrics you will measure, you will want to get a snapshot of the current state to compare to the post-launch state to verify any metric increase or decrease.
- Data infrastructure tutorial – Learning your company’s experimentation and database infrastructure will seem like a daunting task. Your Data Scientist can assist you in:
- Understanding all of the data sources available to you and how to access them – For instance, what portal gives you access to experiment results? Which portal gives you access to time-mapped values for team metrics? Where can you go if you wanted to get your hands dirty with SQL and which databases do you have access to?
- Understanding the experimentation tool -Your experimentation tool may not be the most intuitive to understand. You have to ensure the experiment control and treatment groups are defined correctly and that all other experimentation demographic values are set up correctly.
- Product Metric validation and consultation (Post launch) – After product launch, your data scientist can help make sense of any confusing results. They have a solid foundation in statistics, so they can give insight into whether certain results are valid or should be taken with a grain of salt. In the case an unexpected metric is affected, they will help you build a case for why this result occurred.
- Provide ongoing trend analysis in platform user behavior. Guess what? You have 3 products you’re coordinating with 3 different sets of engineers, designers, and marketers and you may not have the time to analyze current behavioral trends occurring in the ecosystem. Additionally, you weren’t hired for your SQL skills, so you probably couldn’t provide an adequate deep dive analysis even if you wanted to **shrug**. Your data scientist can assist by providing reports on how current user behavior is affecting the core metrics for your platform/product and uncover trends with a level of expertise that requires more than a basic grasp of SQL SELECT and WHERE statements. Their analysis on trends in user behavior can give suggestions on ways to facilitate positive user behavior or reverse negative behavior. This analysis can help spark ideas to improve your current products or develop a completely new product.
- Assist with random data requests – FINALLY, what you thought the data scientist main role was: random data requests. Do you need to know how many users in Canada have filled out the last name field or clicked the “Follow” button between May 1, 2020 and August 20, 2020 at 1PM everyday on an iPhone 8 running iOS 12.2? Your Data Scientist can help you with that. My personal opinion is that I don’t think they enjoy these requests that much, but it’s a tough job and somebody’s got to do it. Well, they’re the only ones that can do it but damn do they make it look good!
Your data scientist is as much of a discoverer of information and problems as you are. They help turn a daunting amount of data into actionable and understandable insight.
The P-Zero PM Tweet This
How to continue to cultivate this relationship?
- Be patient when requesting data. Upon receiving a request, your data scientist must sift through many datasets to find the exact data point you need. Now add your request to the many others they’re getting from various stakeholders in addition to completing their other tasks mentioned above. Yeah, relax.
- Be open to getting your hands dirty with SQL – “Getting your hands dirty” could mean writing your own basic queries, which would require you to know names of all of the tables and their respective attributes. Getting your hands dirty could also mean simply asking your data scientist to send you the SQL code and you can execute it yourself. This will transfer the burden of executing the code and retrieving and organizing the data in a presentable fashion from the data scientist to you. This saves your data scientists time (which I am sure they’ll appreciate) but also gives you an opportunity to understand the schema of your infrastructure a bit more. SQL may seem intimidating at first, but trust me, once you start to pick it up, you’ll realize it reads just like a sentence. Mode has an absolutely amazing SQL tutorial that I highly recommend for all levels of learners.
- Involve them early in your product metric definition process. Perhaps your product requires a metric that isn’t easily trackable given the current infrastructure. You want to be able to track your product’s success, right? Make sure you give your DS team an early heads up on the product and the potential metrics to ensure any infrastructure changes are planned for well in advance.
- Attend as many DS insight deep dives as possible. This may sound extremely nerdy (blame my engineering background), but my favorite meeting of the week is the Data Science deep dive. It is the perfect opportunity to learn more about the process the data experts go through to uncover trends in user behavior. You also get an opportunity to learn basic to advanced statistics concepts. Recently during a data science deep dive, I learned about the Simpson’s Paradox. Fascinating material! If your data science team doesn’t do this on a cadence, I highly encourage you to encourage them to do so!
- Spend time familiarizing yourself with the overall data infrastructure. This is a continuation of #2 above, but the more data you can pull yourself, the more your data scientists will love you because they can focus on the more complex tasks, and the more versatile you will be as a PM. Familiarize yourself with your team’s metric dashboards, which shows the current status of the most important team metrics. Also, familiarize yourself with how to configure these dashboards to uncover the data you need in the format you need it in. Do not get frustrated because familiarizing yourself with the data infrastructure will take some time. One tip is to take notes on everything you learn about how to access/use the data infrastructure. Another one is to bookmark all internal pages which give insight into how to use these same portals.
In Summary...
- Data Scientist’s role? The data scientist is a versatile member of the Prod Squad™ that enables you to integrate data as seamlessly and efficiently as possible into your everyday product decision making.
- How often will you interact with the Data Scientist? If I had a penny for every message I sent to a data scientist, I’d have approximately 1,348 pennies (give or take). Use their expertise early and often because it will make your life 10 times easier.
And here’s another comic to conclude the article….
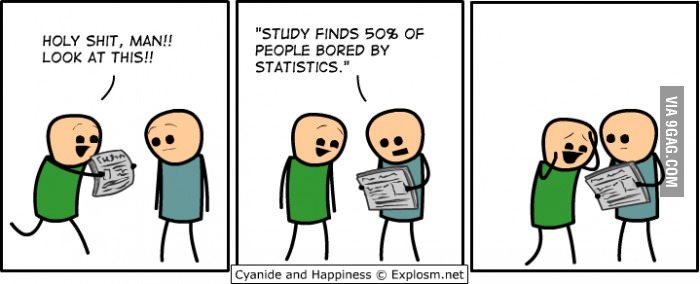
Now that you understand the importance of data scientists, I assume your level of excitement is directly correlated to the fact that you read this article. Right?! Right.
Now go build great products with your new best friend!